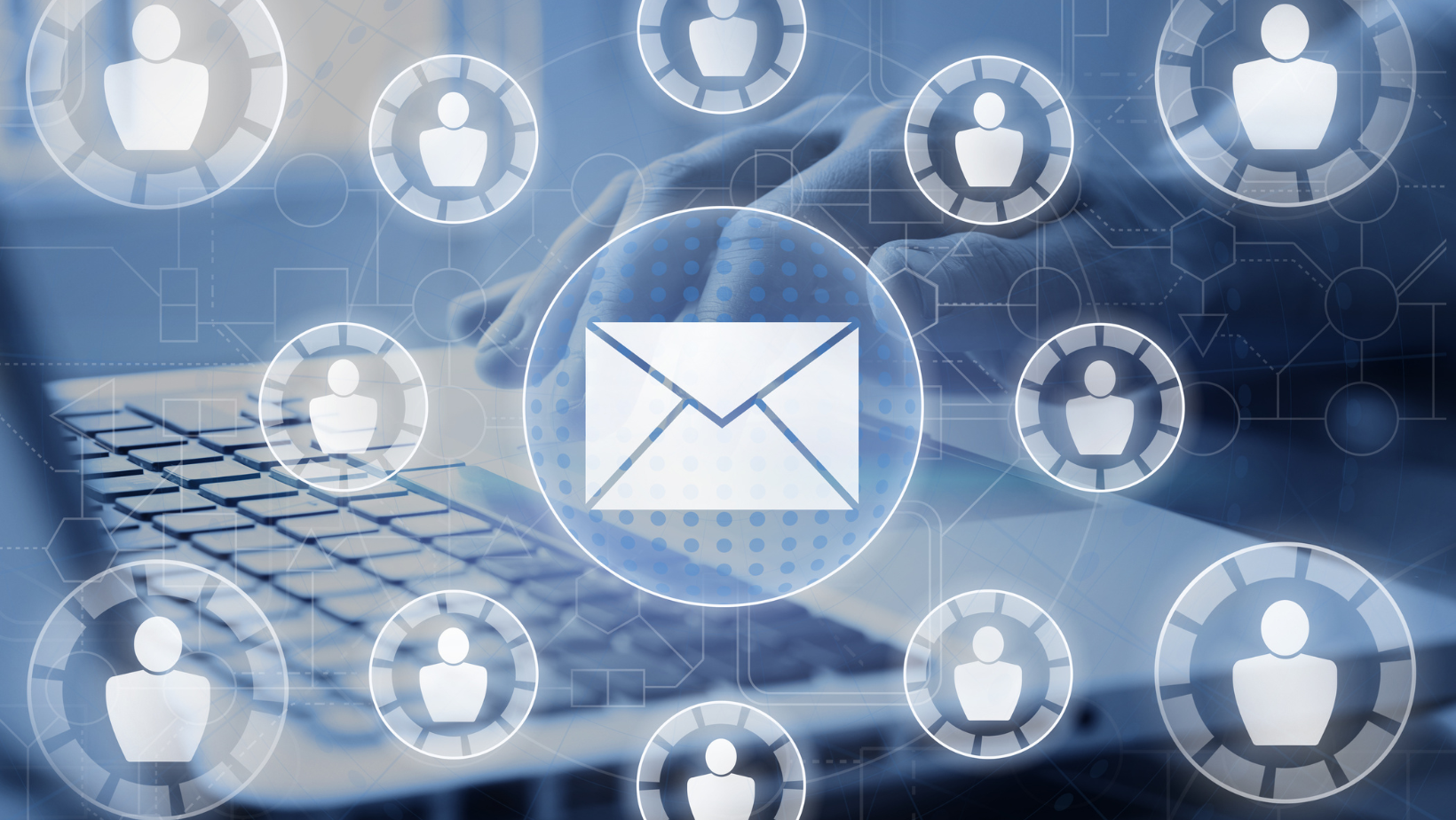
Increased revenue by up to 36% with SabinoDB’s direct mail predictive models
Case summary
“Before using SabinoDB, we spent millions of dollars a year to manage and maintain our direct mail predictive models for our family of apparel brands just to get average campaign results. SabinoDB took a collaborative approach to help us review our models and process. With their help, we were able to improve the accuracy of our predictive models, increase our sales, and reduce the time and cost associated with managing our direct mail campaigns.”
— Chief Marketing Officer
-
Services
DIRECT MAIL PREDICTIVE MODELS
-
Industry
MEN’S & WOMEN’S APAREL
-
Results
UP TO 36% LIFT IN REVENUE
50%+ COST SAVINGS
Client profile
Client is a leading fashion and lifestyle apparel company operating a family of men’s and women’s apparel brands that collectively generate over $1 billion in sales. Client offers its products directly to consumers through its website utilizing digital marketing and direct mail catalogs.
Challenge
Each year, the client mails hundreds of millions of catalogs across its family of brands. The printing and postage costs associated with its direct mail efforts are significant. To achieve an acceptable ROI on its direct mail marketing spend, the client must accurately predict which customers and prospects are most likely to place an order if mailed a catalog and determine who to mail.
Client had historically relied on its in-house predictive analytics team and advanced software tools to build, manage, and maintain a set of state-of-the-art logistic regression predictive models for each of its catalog brands. The datasets used by the client were extremely large comprising multiple years of customer transaction history and highly dimensional spanning multiple channels, websites, demographics, purchase types, and product types. As a result, its process was manually intensive, time-consuming, and expensive. Client was interested in whether a more modern approach using AI and machine learning could yield better results and turned to SabinoDB for help.
Solution
SabinoDB worked closely with client to understand its approach to direct mail predictive modeling and its historical direct mail performance. After developing and testing several different modeling approaches, SabinoDB selected an extreme gradient boosting machine learning model (XGBoost) as the best-performing model in this case. XGBoost is a type of modern machine learning model that was particularly well suited for client’s use case because of its ability to learn from large structured datasets, its speed and scalability, and its overall predictive accuracy.
Results
Using SabinoDB’s machine learning-powered predictive models, client was able to more accurately rank order which customers were likely to respond to a future catalog mailing, increase the total sales generated by its direct mail campaigns, and lower its overall direct mail modeling costs. Key results included:
Increased sales by between 2% to 7% from its current buyer file
Increased sales by between 3% to 36% from its reactivation buyer file
Decreased the costs associated with running and maintaining its predictive direct mailing models by more than 50%